Transforming Businesses with Image Datasets for Classification
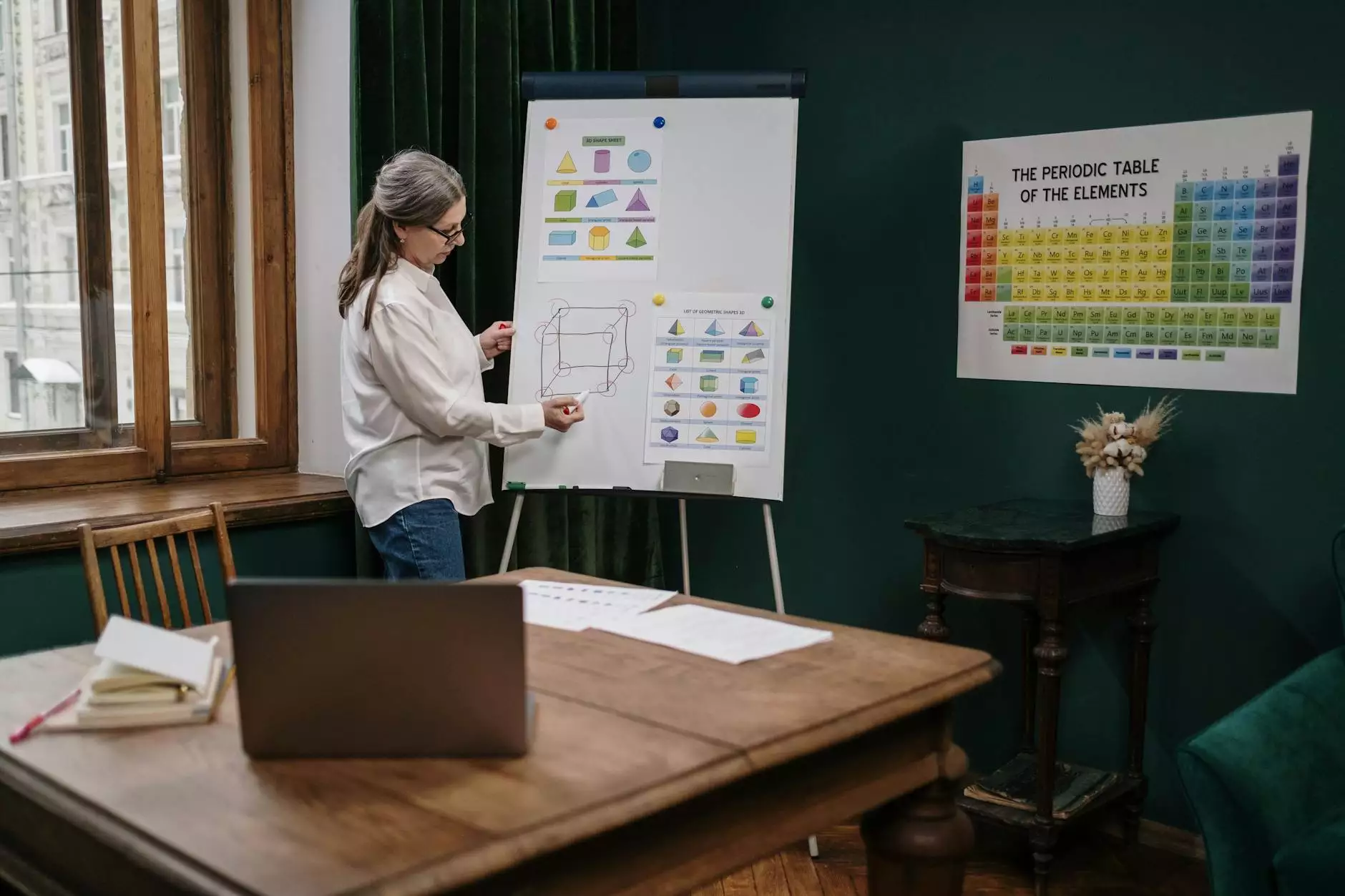
In the rapidly evolving landscape of technology, the significance of image datasets for classification cannot be overstated. Businesses are increasingly recognizing the value of leveraging these datasets to enhance their operations, drive innovation, and maintain competitiveness in their respective markets. This article delves deep into how organizations can harness the power of image datasets for classification through advanced data annotation tools and platforms, paving the way for future growth and efficiency.
The Importance of Image Classification in Modern Business
Image classification plays a pivotal role across a multitude of industries. From healthcare to retail, finance to automotive, the ability to classify images accurately has transformative implications. For instance, in the healthcare sector, deep learning models trained on robust image datasets can assist in diagnosing diseases through medical imaging. In retail, image classification can analyze customer behavior and preferences, optimizing inventory management and marketing strategies.
Understanding Image Datasets
At its core, an image dataset is a collection of images that are utilized for training machine learning models. These datasets consist of multiple categories and are labeled accordingly, making them essential for classification purposes. The quality and quantity of these datasets directly influence the model's accuracy and efficacy.
Characteristics of High-Quality Image Datasets
- Diversity: A well-rounded image dataset incorporates a wide range of categories, contexts, and environments to ensure models are trained on varied examples.
- Labeling Accuracy: Each image must be meticulously labeled to reflect its content accurately, which is crucial for the success of classification algorithms.
- High Resolution: Higher quality images facilitate better feature extraction, enabling the model to learn efficiently.
- Size: Larger datasets play a significant role in improving model performance, as they provide more data points for learning.
How Data Annotation Tools Elevate Image Classification
The advent of sophisticated data annotation tools has simplified the process of creating and managing image datasets. These tools allow organizations to annotate images efficiently, ensuring that the resulting datasets are not only large but also precise and tailored to specific use cases.
Key Features of Effective Data Annotation Platforms
- User-Friendly Interface: A good data annotation platform should be intuitive, allowing users to annotate images without steep learning curves.
- Collaboration Tools: Features that facilitate collaboration among team members streamline the annotation process, yielding faster results.
- Quality Control Mechanisms: Automated checks or manual review processes ensure the accuracy of labels, which is critical for training reliable models.
- Scalability: Platforms should be able to handle large volumes of images efficiently, supporting business growth and adaptation.
The Benefits of Using Data Annotation Tools for Image Datasets
Implementing data annotation tools to create image datasets for classification provides several benefits, including:
- Increased Efficiency: Automation and streamlined processes reduce the time taken to create datasets.
- Improved Accuracy: Advanced annotation tools enhance the precision of labels, leading to higher-performing models.
- Cost-Effective Solutions: Reducing manual effort lowers costs associated with data preparation, making it a smart financial decision.
Best Practices for Building Image Datasets
Constructing an image dataset for classification is both an art and a science. Here are some best practices to consider:
1. Define Clear Objectives
Before you start collecting images, it’s crucial to define the goals of your classification task. Are you identifying objects, classifying images into categories, or detecting anomalies? Having clear objectives guides the annotation process.
2. Collect Diverse Data
Gather images from various sources to ensure diversity. This could include public datasets, proprietary collections, or user-generated content. Ensure that the images reflect the real-world scenarios your model will encounter.
3. Implement Rigorous Annotation Protocols
Develop protocols that your annotators can follow to maintain consistency and accuracy in labeling. This may include detailed guidelines and reference images to illustrate various categories.
4. Leverage Technology
Utilize advanced annotation tools that incorporate features like bounding boxes, segmentation, and keypoints. These tools not only speed up the process but also enhance the granularity of your annotations.
5. Conduct Regular Quality Checks
Quality assurance is paramount. Implement regular checks to review and validate the annotations. Feedback loops where annotators can learn from feedback improve overall quality.
Case Studies Showcasing Success Through Image Classification
Several organizations have successfully implemented image classification to enhance their business processes. Here are a few notable examples:
Healthcare: Early Disease Detection
In the healthcare sector, a leading medical imaging company revolutionized its diagnostic tools by utilizing high-quality image datasets. By annotating thousands of medical images for training deep learning algorithms, they significantly improved early detection rates of diseases such as cancer, allowing for timely intervention.
Retail: Personalized Shopping Experiences
Global retail giants have tapped into image classification to analyze customer preferences. By collecting and classifying images of products and customer interactions, they optimized inventory and personalized marketing strategies, which resulted in increased sales and enhanced customer satisfaction.
Automotive: Enhancing Safety Features
In the automotive industry, manufacturers incorporated image classification models to enhance driver-assistance systems. By training models on extensive annotated datasets, they developed features like lane keeping assistance and object recognition, thereby improving safety on the road.
The Future of Image Classification in Business
The future of image datasets for classification is bright as businesses continue to embrace AI and machine learning technologies. With advancements in deep learning and computer vision, the potential applications are boundless. Companies that invest in high-quality datasets and robust annotation tools will undoubtedly lead the charge in innovation and efficiency.
Conclusion: Empowering Business with Image Datasets
In conclusion, leveraging image datasets for classification is not merely an operational strategy; it is a powerful catalyst for business transformation. By investing in quality data annotation tools and practices, organizations can harness the full potential of image classification, driving growth and fostering innovation.
Businesses today are at a crossroads where the right approach to image classification can set them apart in competitive markets. Those who embrace data-driven decision-making through effective use of image datasets will undoubtedly thrive in the technologically advanced future.